Using prior knowledge to augment data-driven predictions in biomedicine
Machine learning using large-scale Transformer models has many applications in discovery research to enhance our understanding of biochemistry and metabolism. Bio-ontologies include consensus hierarchical classifications of entities in their domain and are a key resource for biomedical data-driven discovery research. While the potential uses of large-scale machine learning models for biomedical discoveries are growing, there are well-known challenges with such models: data for the given prediction problem may be sparse, the model may learn spurious associations that lack generalisability, and the opacity may hinder the utility of the prediction in discovery research where novel findings need to be understood. Incorporating prior knowledge from ontologies can improve the generalisability and robustness of predictive models as well as provide avenues towards more robust interpretability. Through a case study in toxicity prediction from chemical structures, I will illustrate an ontology-based approach to pre-training that improves performance and interpretability of a Transformer network.
Machine learning using large-scale Transformer models has many applications in discovery research to enhance our understanding of biochemistry and metabolism. Bio-ontologies include consensus hierarchical classifications of entities in their domain and are a key resource for biomedical data-driven discovery research. While the potential uses of large-scale machine learning models for biomedical discoveries are growing, there are well-known challenges with such models: data for the given prediction problem may be sparse, the model may learn spurious associations that lack generalisability, and the opacity may hinder the utility of the prediction in discovery research where novel findings need to be understood. Incorporating prior knowledge from ontologies can improve the generalisability and robustness of predictive models as well as provide avenues towards more robust interpretability. Through a case study in toxicity prediction from chemical structures, I will illustrate an ontology-based approach to pre-training that improves performance and interpretability of a Transformer network.
Speakers
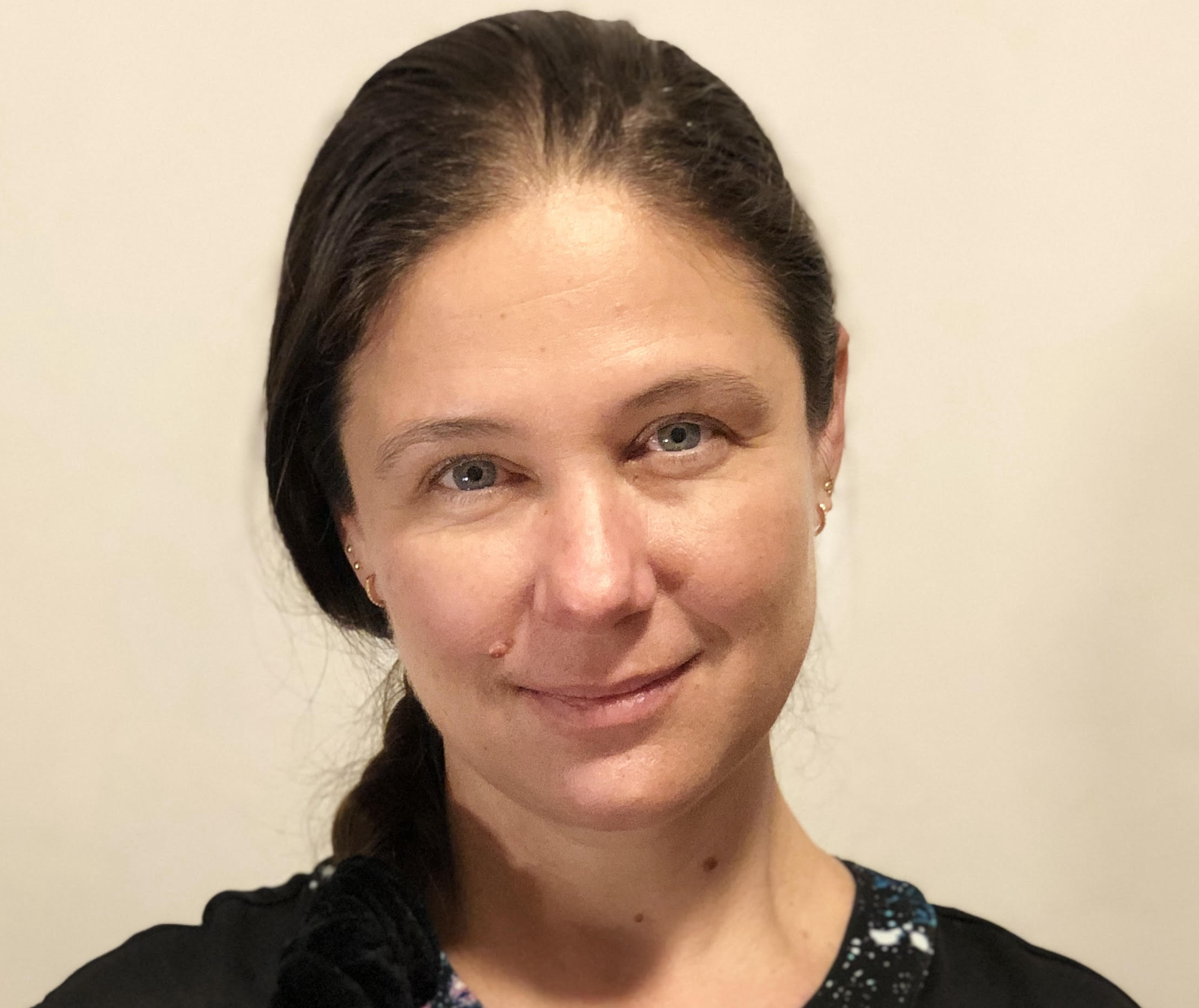
Janna Hastings obtained her PhD in Computational Biology from the University of Cambridge (2019) and holds Masters degrees in both Computer Science (2011) and Philosophy (2012). She is currently Assistant Professor of Medical Knowledge and Decision Support at the Institute for Implementation Science in Health Care, Faculty of Medicine, University of Zurich, and Vice-Director of the School of Medicine at the University of St. Gallen. Her research focuses on medical data science for clinical applications and biomedical discovery, with a special focus on how the powerful data-driven approaches to can be made more generalisable and interpretable through the use of prior knowledge.